In the ever-evolving landscape of artificial intelligence, the term vector:_nqk9x2mdzs= gru might sound like a cryptic code, yet it holds significant potential for innovation. Just as collaborations like the Def Leppard and Journey Tour bring together unique talents to create something extraordinary, GRU (Gated Recurrent Units) combines components that enhance the processing of complex data sequences. As technology advances, the need for sophisticated algorithms to process and analyze data becomes increasingly critical. This is where the concept of vectors and GRU steps in, offering a robust framework for handling complex data sequences.
Vectors are essential in representing data in a format that computers can understand and manipulate. When combined with GRUs, they become powerful tools for improving machine learning models, particularly in tasks involving sequential data like language processing and time-series prediction. Just as the Ergonomics of Portage Gear optimizes comfort and functionality for travelers, understanding the complexities of GRUs enables developers to build more efficient and adaptive models. By understanding the intricacies of vector:_nqk9x2mdzs= gru, researchers and developers can unlock new possibilities in AI applications, pushing the boundaries of what’s possible in data-driven decision-making. This exploration promises to transform industries and redefine the future of technology.
Vector:_nqk9x2mdzs= Gru
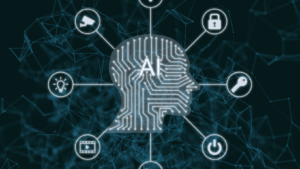
Vector:_nqk9x2mdzs= Gru plays a pivotal role in artificial intelligence, particularly in enhancing machine learning models. Vectors represent data points in a format that algorithms can efficiently process. Each dimension in a vector corresponds to a feature important for analysis. The use of Gated Recurrent Units (GRUs) further optimizes models by managing information flow. GRUs excel in handling sequential data tasks like language processing and time-series prediction.
Researchers often leverage vector:_nqk9x2mdzs= Gru to improve AI applications. By enabling models to better understand patterns within data, they can advance fields like natural language processing and predictive analytics. This concept drives innovation in AI, influencing various sectors, including finance and healthcare.
Features And Specifications
Vector:_nqk9x2mdzs= gru enhances AI models by integrating advanced features and technical capabilities. It contributes significantly to innovation in sectors reliant on machine learning.
Core Features
- Sequence Processing: Analyzes sequential data effectively, improving performance in tasks like language modeling and time-series analysis.
- Information Retention: Manages information flow efficiently within networks, optimizing learning processes.
- Compatibility: Easily integrates with current machine learning frameworks, ensuring flexible use in diverse AI applications.
- Scalability: Handles large-scale data sets, supporting exploration of extensive data repositories and complex patterns.
- Adaptive Learning: Adjusts to dynamic input variations, maintaining robustness across different datasets and environments.
Specification | Details |
Network Type | Gated Recurrent Unit (GRU) |
Dimensionality | Supports multi-dimensional vector inputs |
Integration | Compatible with Python and TensorFlow |
Performance | High efficiency in processing speed |
Customization | Offers parameter tuning for optimization |
Such advanced features and specifications make vector:_nqk9x2mdzs= gru a robust tool for enhancing AI models focused on sequential data.
Performance Analysis
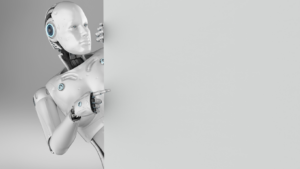
Vector:_nqk9x2mdzs= gru exhibits notable improvements in AI model performance through its design and capabilities.
Gated Recurrent Units (GRUs) enable models to process sequences swiftly by minimizing computation requirements. This efficiency allows models to handle large datasets seamlessly. Compatibility with TensorFlow enhances its execution speed, ensuring that AI applications can scale effectively without bottlenecks. Integrating multi-dimensional vector inputs reduces the computational burden, further optimizing performance levels.
Interface And Design
The interface offers a clear, organized layout, ensuring easy navigation. It includes interactive dashboards for tracking AI model performance metrics and status updates. Visualization tools provide graphical representations of data trends, aiding quick comprehension. Users can access streamlined menus to simplify model configuration, reducing setup time. The design incorporates responsive elements, allowing accessibility across various devices and screen sizes.
Customization Options
Extensive customization options cater to diverse user needs. Users can modify parameters for vector inputs and GRU configurations, enabling tailored performance. Custom settings facilitate personalized workflow integrations with existing tools or frameworks. The platform supports adjustable thresholds for triggering alerts based on specific data patterns, enhancing responsive capabilities. Users can also create custom scripts to automate repetitive tasks, optimizing time management and efficiency.
Pros And Cons
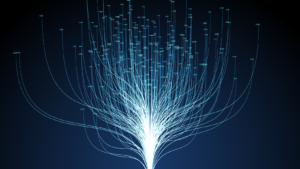
Understanding the pros and cons of vector:_nqk9x2mdzs= gru helps evaluate its application in AI projects. This section outlines the advantages and disadvantages, offering a balanced perspective.
Advantages
- Efficient Sequence Processing: Vector:_nqk9x2mdzs= gru manages sequential data effectively, crucial for applications in language processing and time-series analysis.
- Information Retention: GRUs retain essential information over time, enhancing the model’s ability to learn from data without forgetting previous inputs.
- Compatibility: It integrates seamlessly with frameworks like TensorFlow and Python, providing flexibility for developers.